In the previous blog post we have talked about basic principles of building AI assistants. Let’s take them for a spin with a product case that we’ve worked on: using AI to support enterprise sales pipelines.
B2B sales in the enterprise world are very lucrative, once they go through. However, they can take years to settle. This means, that a company needs to have multiple leads in the sales pipeline at once. This is a resource-intensive process that is frequently limited by a human factor; sales become the bottleneck.
It is possible to remove the bottleneck from sales if we could:
One project that we have worked on, aims to do exactly that. It is an AI assistant that ingests publicly available information about large companies: Annual Reports, SEC Filings or ESMA documents. This is a large set of data, potentially filled with really good leads.
We just need to sift through that data, finding information about companies and prioritising cases according to our unique sales process. How hard can that be?
Exploring hallucinations of AI assistants
As it turns out, classical vector-based AI RAG systems fail even at the most simple sales question: “Which company has the most cash available right now?”
In theory, getting this answer from an annual sales report is as easy as looking for the “Cash” entry in the “consolidated balance sheet”.
As you can see in our Enterprise AI Leaderboard section (from the February LLM Benchmark) even the best document retrieval systems sometimes fail to answer questions about a single annual report:
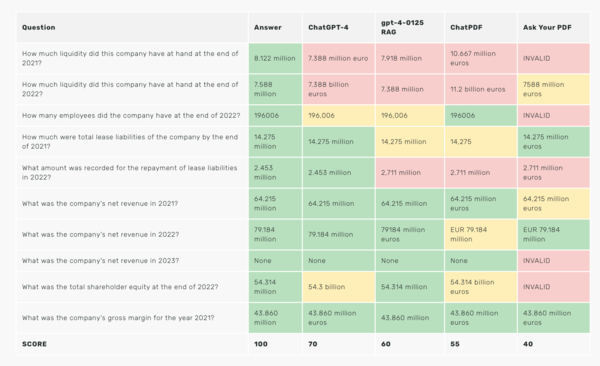
Things get substantially worse, if you would try to upload multiple annual reports and ask “Which company has the most cash”.
Here is an experiment you can reproduce with an AI assistant of your choice:
Get annual reports for 2022 from Christian Dior, Bellevue Group and UNIQA Insurance Group (or any other combination for that matter)
Upload these to a RAG
Ask a very specific question:
You are CFO-GPT. Quickly answer, which of the companies has more liquidity right now? And how much? Don't make up information, if you are not certain.
At this point, it looks like the system should either give us the name of the company with the numeric value OR give up, right?
So what would ChatGPT-4 (still the best in its class) do?
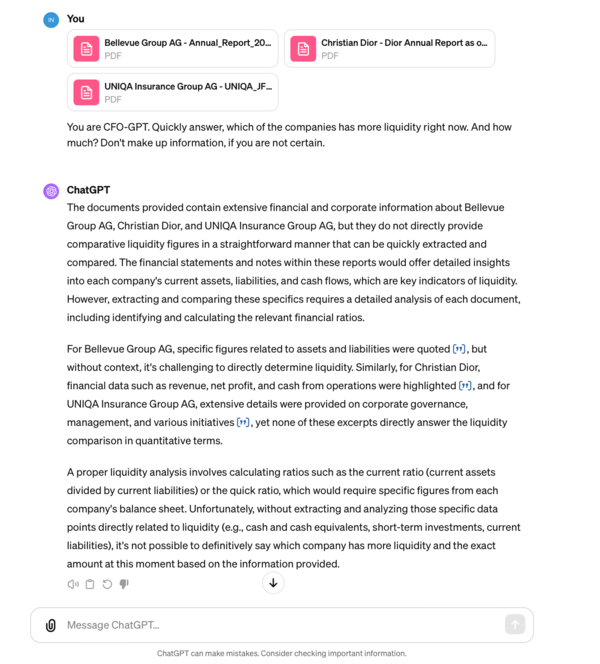
What if we take LlamaIndex which promises to “turn your enterprise data into production-ready LLM applications”?
Answers will be more concise but spectacularly useless:
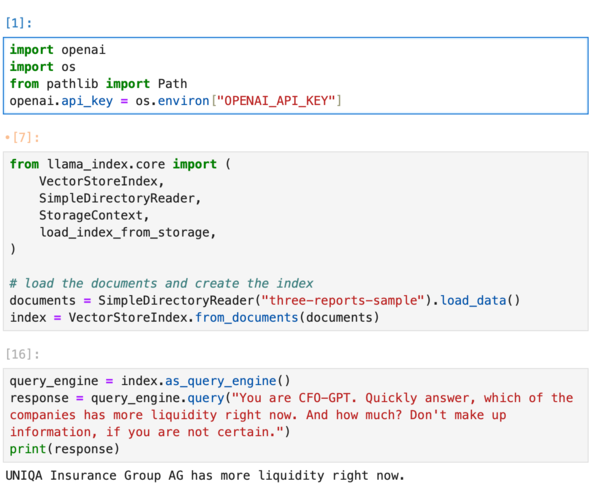
Ask it a couple of times and it will keep on coming up with creative ideas:
- UNIQA Insurance Group AG has more liquidity right now compared to Bellevue Group AG.
- Bellevue Group has more liquidity right now.
- UNIQA Insurance Group AG has more liquidity right now. The total financial liabilities due within 3 months for UNIQA amount to €12,897 thousand, while Bellevue Group AG has CHF 26,794 thousand due within the same period.
Proponents of vector-based RAG systems will say that nobody uses just LangChain or LlamaIndex and that you should build a dedicated assistant on top of these frameworks first. Although that is a bit of moving the goalposts, you can still independently verify a system like that. Just take a couple of annual reports (the more - the merrier), upload them and ask questions like the ones provided above.
If you think your system will pass such a test, I would be glad to test it personally and share the results publicly. We have 50GB of annual reports to use as test data!
Applying Domain-Driven Design to the problem
There is actually an easy way to build a system capable of answering trivial questions like that. It starts with two simple steps:
How would a real domain expert approach this problem? He would probably know how reports are structured and would look for any lines mentioning liquidity or cash flow in the consolidated balance sheets.
We can just replicate this approach. Instead of shredding all documents into tiny pieces and putting them into the vector database, we can extract information from the documents into a knowledge map.
Just like it is depicted at the bottom path in this image:
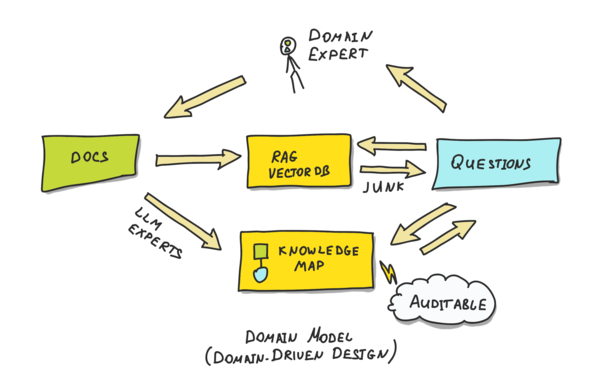
We have documents on the left. There are LLM extractors (experts) that go through these documents, find bits of information and populate the knowledge map in advance. The knowledge map is designed in such a way that when the question to an AI assistant comes, answering it becomes a simple task.
Unlike the contents of embedding vectors and graph nodes, this knowledge map will be auditable and easily readable by non-technical people.
If all our system has to do is to talk about liquidity and compare different companies on that topic, then this knowledge map could be represented by a single object that fully fits into the context of a large language model:
Bellevue Group
2022 Liquidity: 64,681,000 CHF
2021 Liquidity: 84,363,000 CHF
UNIQA Insurance Group AG
2022 Liquidity: 667,675 EUR
2021 Liquidity: 592,583 EUR
Christian Dior
2022 Liquidity: 7,588 million EUR
2021 Liquidity: 8,122 million EUR
2020 Liquidity: 20,358 million EUR
Larger knowledge maps will need specialised storage systems that can be queried by a LLM during the prompting. It is not uncommon to see knowledge maps with hundreds of thousands of entities.
Fortunately, ChatGPT is quite good with SQL, NoSQL and even pandas. This is one of the reasons why we are tracking the "Code" column in our LLM benchmarks.
Given a knowledge map like this, it feels like cheating to ask ChatGPT questions. Pass it together with the prompt:
You are CFO-GPT. Quickly answer, which of the companies has more liquidity, and how much? Don't make up information, if you are not certain."
The answer will be very precise, and it will not change between runs:
Christian Dior has the highest liquidity in 2022 with 7,588 million EUR.